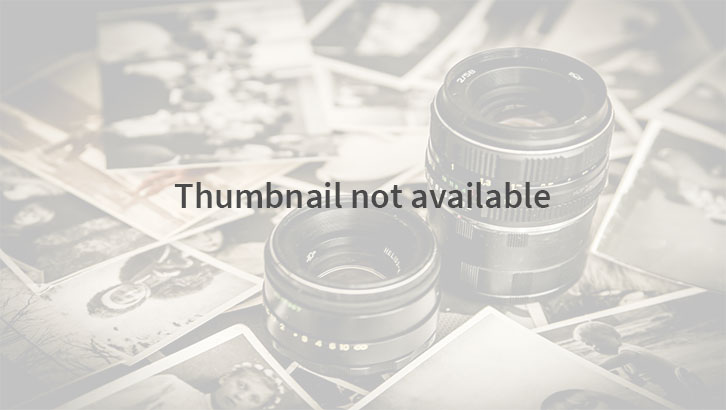
2012-8 Meta-analysis of a rare-variant association test
Genome-wide assocation studies have often been carried out by meta-analysis rather than by pooling individual-level data. For one-dimensional parameter estimates and the corresponding tests of …